A graph-based database of hierarchical brain features
Noah Lee (Columbia University), Arno Klein (Columbia University)
Studies of brain morphometry have been predominantly restricted to extremely limited characterizations of regions of interest, such as their volume, cortical thickness, and surface area. Recognizing that there exist algorithms for extracting anatomical structures within brain images as well as rich shape descriptors, we set out to describe the shapes of brain structures and make the data publicly available for meta-analyses, to provide points of comparison, for training or testing algorithms and hypotheses, and to inform automated anatomical labeling software that we are developing. To this end, we are assembling the first online database containing quantitative shape information about individual structures in manually labeled brain images. The Mindboggle database will be hosted on http://www.mindboggle.info.
The publicly available brain images underlying our database will initially consist of hundreds of adult T1-weighted MRI volumes whose sulcal/gyral anatomy have been manually labeled by experts (Neuromorphometrics, MA). We will automatically extract brain structures from these labeled images and organize them in a nested hierarchy, where pits are points within a fundus, which is a curve on the edge of a ribbon, which is the medial surface of a basin, which is a volume within a fold of the brain, with folds themselves organized hierarchically. The database will contain geometric, shape, and spectral information as features characterizing each structure in a local-to-global manner, as well as their relationship to other structures and labeled regions. Because the features relate to nested, hierarchical structures, we have selected a noSQL (not only SQL) database using a graph-based data model as opposed to a standard relational database because we want (1) our feature database to be flexible enough to accommodate changing data and knowledge representations, (2) to naturally represent and store connected and semi-structured data, and (3) to support large-scale storage while also exploiting the power of graph inferences to perform feature analyses in populations.
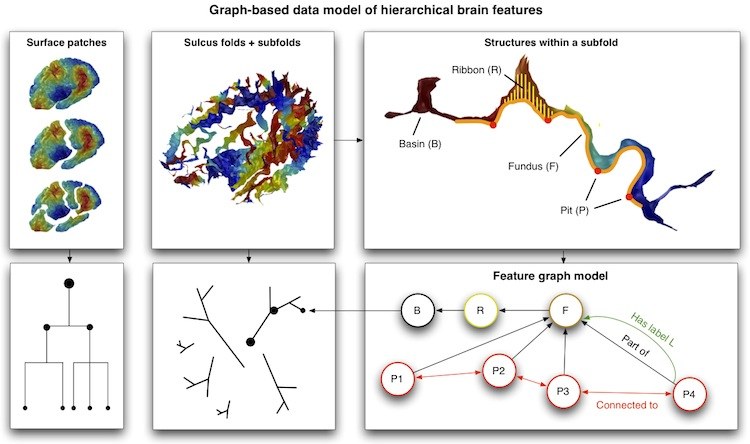