Spike train statistics of simulated and in vitro data
Kerstin Lenk (Lausitz University of Applied Sciences), Barbara Priwitzer (Lausitz University of Applied Sciences)
Very few models to date have been developed to examine inhibitory and excitatory effects as observed in in vitro neuronal networks. In such in vitro experiments frontal cortex tissue of embryonic mice is cultivated on a multielectrode array (MEA) neurochip [1]. Action potentials are derived from the electrodes of the MEA neurochip. The temporal occurrence of the action potentials is recorded as time stamps. The object for the present work was to simulate experimental data and to compare the simulated data with MEA data using statistical methods.
We developed a spiking neuronal model. The model called INEX (inhibitory-excitatory) is a high-dimensional cellular automaton whose cells represent neurons with two possible states: ON or OFF. The binary model has several characteristics: neurons are active without external input or stimulus as observed in experiments. This is realized by the assumption that the spikes obey a Poisson distribution. Full connectivity of the network (with direct feedback, i.e. neurons receive their own output) implies that the activity can be written as an inhomogeneous Poisson process. The inhomogeneity of the neuronal activity is realized by inhibitory or excitatory synapses of varying strength. The corresponding parameters are called weights. Spike time history is added, i.e. the probability of spike occurring increases following a spike in the previous time slice. The outcome of the model are time stamps of spikes for each neuron.
For the simulation, a network with 10 neurons ran for 30 minutes with time slice Δt = 5 ms. This choice of Δt ensures that the refractory period of real neurons is reflected in the model. Nine inhibitory neurons with synaptic weights between -0.2 and 0 and one excitatory neuron with synaptic weights between 0 and 0.7 were generated. For the resulting spike train, spike and burst describing parameters were calculated [2]. The spike train data of one selected neuron was binned with a width of 5 seconds. This binning process reduces uncertainties and enables us to examine the average behaviour of a dataset. The following statistical methods were applied (described in [3]) in order to compare the simulated data with experimental MEA data: 1) A box plot of the binned and sorted INEX data shows their distribution. 2) The spikes per bin were plotted as a histogram against their count. 3) The INEX data was plotted against a Poisson distribution in a QQ-plot. The same three statistical methods were applied to a 30 minutes spike train obtained from an in vitro MEA experiment. Additionally, the binned INEX data was plotted against the binned MEA data in a Q-Q plot (figure). The above described statistical methods were applied to ten single neurons.
As a result of our work we can summarize that the INEX model shows potential to simulate the neuronal activity which can be also observed in experiments with MEA neurochips. The described statistical methods showed that the INEX data resemble the MEA data.
References
[1] Johnstone AFM, Gross GW, Weiss DG,
Schroeder O, Gramowski A, Shafer TJ: Microelectrode arrays: A physiologically
based neurotoxicity testing platform for the 21st century. NeuroToxicology
2010, 31: 331-350.
[2] Schroeder OHU, Gramowski A, Jügelt K,
Teichmann C, Weiss DG: Spike train data analysis of substance- specific network
activity: Application to functional screening in preclinical drug development.
6th Int. Meeting on Substrate-Integrated Microelectrodes, 2008.
[3] Kass RE, Ventura V, Brown EN:
Statistical Issues in the Analysis of Neuronal Data. Journal of Neurophysiology
2005, 94: 8-25.
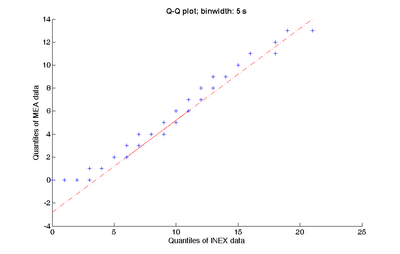