Understanding Human Implicit Intention based on EEG and Speech Signals
Suh-yeon Dong (Department of Electrical Engineering and Brain Science Research Center, KAIST), Daeshik Kim (Department of Electrical Engineering and Brain Science Research Center, KAIST), Soo-Young Lee (Department of Electrical Engineering and Brain Science Research Center, KAIST)
Abstract
There are many approaches to understand human intention. The objective of human computer interface (HCI) research is to make machine that understands human intention with high accuracy. In the future machine will be able to understand human intention and communicate with him/her as same as human-human communication. Previous studies mainly concentrated on interpreting human’s intention expressed explicitly. Of course we show our intention by speech, gesture, and facial expression, etc. However, these explicit expressions may not be enough to understand what we really intend to. Moreover, we sometimes do not show our intention explicitly. There are many situations not to disclose our minds. If machine learned only interpreting explicit expression like erstwhile studies, it is not possible to ensure the accuracy.
Ten healthy volunteers were studied. The participants read obvious and non-obvious sensitive sentences(Table 1.) and indicated their agreement or disagreement by their speech during recording 32-channel electroencephalography (EEG) along their scalp. The obvious sentences consist of two groups, i.e., one for the obvious ‘agreement’ and the other for obvious ‘disagreement’. The ‘agreement’ or ‘disagreement’ on non-obvious sentence is subject dependent. To create situations of discrepancy between the explicit intention and implicit intention, the non-obvious sentences consist of sensitive personal questions to which human subject may not want to answer correctly. We assumed these sentences may be related to their private life. And it is also assumed that brain activation may not be the same when people expresses differently from their real intention.
ICA on EEGLAB was used to identify and remove eye movement artifacts. And power spectra are averaged over 6 standard EEG frequency bands of delta (2-4 Hz), theta (4-8 Hz), alpha (8-13 Hz), beta1 (13-20 Hz), beta2 (20-35Hz), and gamma (35-46 Hz). First, the obvious situation was examined. We found that the classification results with nonlinear support vector machine (SVM) for prefrontal EEGs showed significant differences between agreement and disagreement situation. Secondly, the prefrontal EEGs for the non-obvious situation were tested by the SVM classifier trained with the EEGs of obvious situation.
We also trained an SVM for speech signals from the obvious sentences, and tested the speech signals from the non-obvious sentences. Since no ground truth labels were available, the SVM outputs for corresponding EEG were considered as the labels of implicit intention. The results showed that the classifier outputs for speech and EEG signals have high correlation. It may be used to understand human implicit intention, which is different from the explicit intention.
Keywords
Human implicit intention; Electroencephalography; support vector machine; EEGLAB;
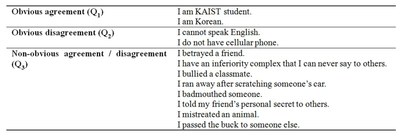